How do foundation models improve decision making?
Foundation models have a great potential to help in decision making, providing insight that can help in various situations, including multiple use cases across an enterprise, as they are trained on a huge amount of data from multiple sources.
One use case in business setting would be analysing and interpreting large amounts of unstructured data, such as customer reviews and survey responses that can help decision makers gain insights and make better data-driven decisions. To determine consumer sentiment around goods or services, foundation models like BERT can examine reviews, comments, and social media posts, with high accuracy rate, providing market insights in an efficient manner. This information can then be further used to assist with product development and customer service and marketing strategies.
Furthermore, foundation models can process and analyse huge amounts of data and identify patterns and trends – an unfeasible task for humans – thus giving decision makers a better understanding of the available and highly useful data. To demonstrate with our own example – Amygda’s time series foundation model can be used for multiple use cases, including smart maintenance, route optimisation, or even equipment life forecasting, helping decision makers in aerospace and rail gain an insight into the factors and reasoning behind the decision making process.
However, even though foundation models have a great potential for assisting in decision making in various business cases across industries, at the moment AI model application remains very narrow – mostly in generative AI, such as in content creation. Foundation models can be expensive to access and require a significant amount of computational power to run effectively, posing a challenge for businesses that do not have the resources to adopt these technologies.
This is an indication that we may be at an inflection point when it comes to the adoption of foundation models across industries. Emerging start-ups are penetrating the industrial sector to help with decision making in industries that can highly increase their ROI by having better insight from equipment that produces a large amount of data (e.g. sensor data). The problem that foundation models solve is simple – one model, multiple use cases, instead of one model, one use case.
Problems with foundation models and how to overcome them
According to a paper by Google Research, Brain Team et al. on foundation models and decision making, new challenges are emerging, such as how to perform long term reasoning and planning, as when these models are deployed in real work environments, they interact with external entities, exposing the model to an ever-larger dataset curated for multimodal and multitask interaction.
To overcome this challenge, foundation models and decision-making should be working jointly, as in the example of using human feedback for dialogue tasks in decision making. Foundation models may not completely replace the need for experts, but they allow organisations to utilise human experts for the most crucial findings at the right moment. That’s why we advocate for the integration of human and AI efforts.
Interpretability remains a challenge in adopting foundation models, as they can be difficult to understand, limiting the potential of the models. To overcome this challenge at Amygda, we combine human and AI input equally to create explainable and trustworthy models by the use of explainability algorithms sitting alongside our solution.
Moreover, data quality used to train the models is of crucial importance, as it can bring about inaccurate outcomes or insights, perpetuating biases. We try to overcome these using unsupervised and semi-supervised techniques, with further development focusing on self-supervised learning.
Can start-ups create good ML foundation models considering the lack of data?
Acknowledging the lack of data on the end of start-ups is an indication that indeed remains a challenge, but fortunately it can be overcome in collaboration with companies that start-ups work with. Taking the example of the aerospace industry, data is gathered using sensors placed in various locations, for example in an aircraft engine to check performance. The problem these organisations have is not the lack of data, but instead the abundance of it and finding the proper techniques to interpret it in order to make a decision.
Our experience working with a leading US airline, to determine the component at fault causing long-term issues with the Hydraulic Systems on aircraft, shows the lack of data can be overcome by combining it with whole fleet data accompanied by maintenance information logs.
Building on the experience, collaboration and cooperation with other organisations that use our models to interpret the data which helped detect failures, the lack of data is a challenge that can be overcome. Foundation models are built to learn on various data and be implemented on multiple use cases, thus we build on our experience, knowledge and data every time we interact with a new customer. It has a network effect, creating better insights for customers and learning opportunities for our foundation models.
What is the main focus for Amygda’s AI Lead and team to build in the aerospace sector?
We have done a lot of work on unsupervised and semi-supervised capability, which can withstand data-centric challenges. This approach has allowed us to quickly solve novel problems, regardless of industry or specific equipment. We have used large amounts of data to solve particular cases, but recently we’ve shifted our focus towards leveraging this data to its fullest potential.
Our goal is to build an intuitive understanding of the underlying mechanics of the equipment, while identifying any gaps in our knowledge. Ultimately, we want to create a pre-trained foundation model that can be used for transfer learning in a specific use case. This is our current focus within the aerospace sector, and we are working at full steam ahead to achieve our goal.
As part of our work, we are generating synthetic data from a variety of aerospace industry configurations and combining it with actual industrial data. We are using this data to train large transfer learning-based models, testing different deep learning architectures and validating them with specific use cases in specific subdomains. Our goal is to create a step-change in how we serve our customers by delivering reusable and flexible AI solutions in the aerospace sector, and beyond.
Market opportunity for foundation models
Although it is difficult to determine the market opportunity for foundation models in the industrial sector, some preliminary analysis on generative AI from Acumen Research and Consulting shows that the estimated value of the global generative AI market size in 2021 is $7.9bn, while in 2030 it is expected to be at $110.8bn. A report by IBM Global AI Index in 2022, shows that only 35% of companies reported using AI in their business. Ark Investment Management expects that $80 trillion in enterprise value will be created using AI by 2030, around $25trillion directly linked to foundation AI models.
All in all, this represents a great opportunity for businesses to adopt AI at scale, to make better decisions and the best use of their data. With new challenges come new opportunities, and the opportunity in the data-driven economy is to increase ROI by interpreting the abundant data that is ever increasing, but not explainable by humans.
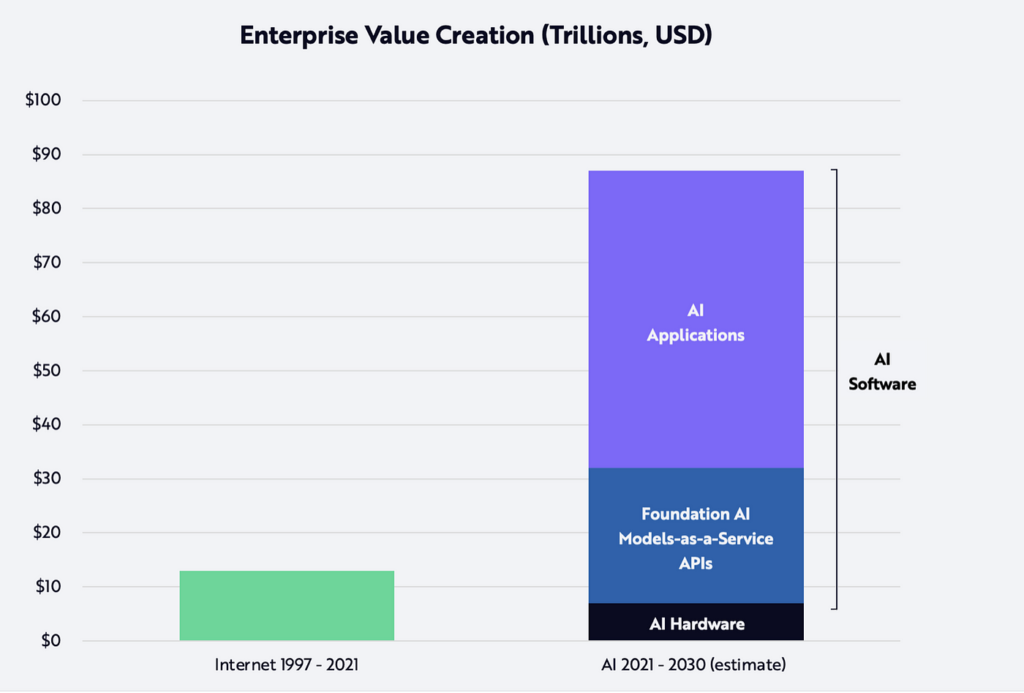
Drop us a line at [email protected] to chat to us about how we can leverage our existing solutions for your fleet of assets.