Time series foundation models for sensor data
Reduce the time for each new use case for all assets in your fleet from months to weeks (and soon to days), using flexible and reusable AI models.
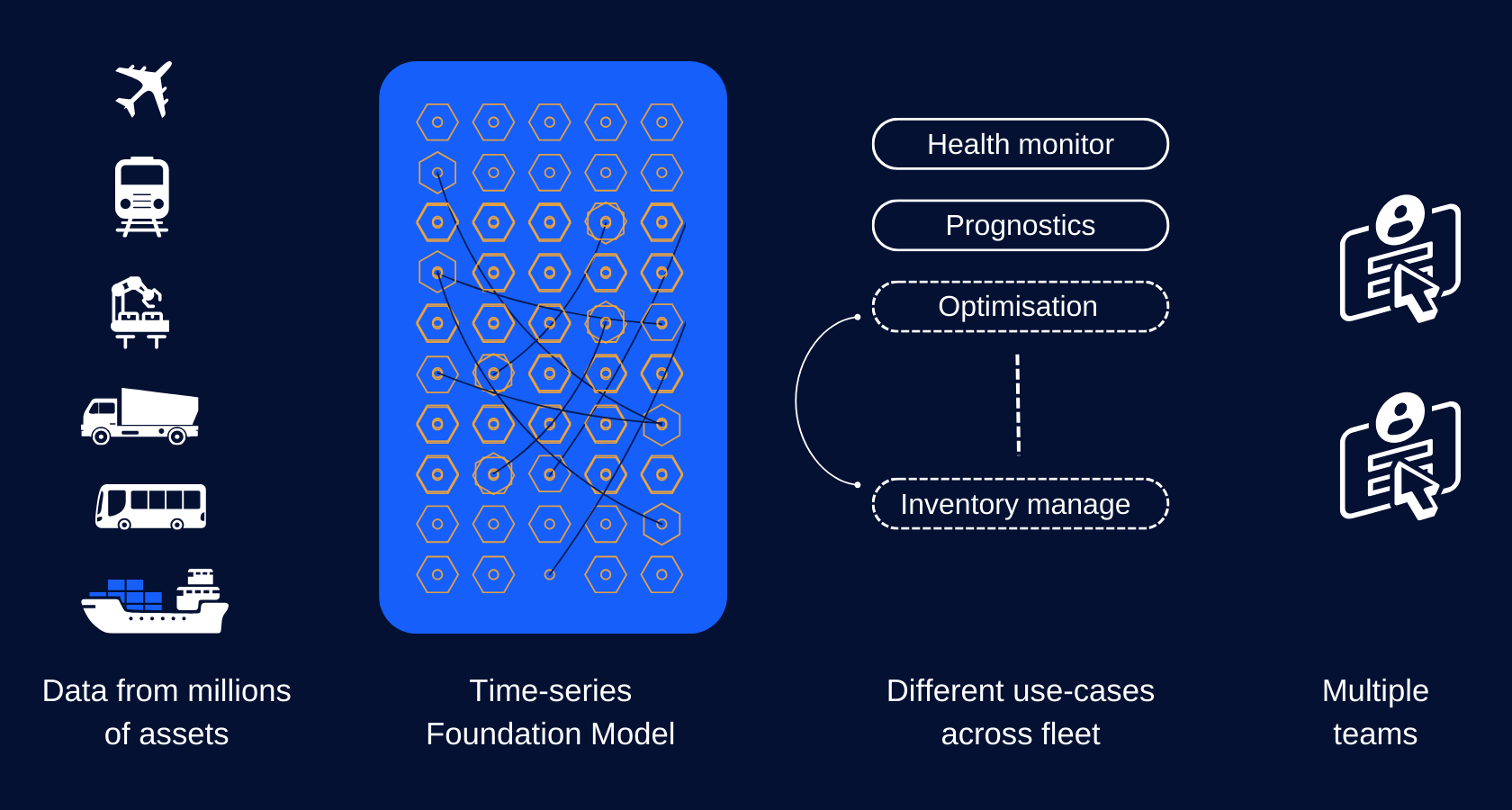
The Problem
Models for each use case take 4 to 6 months to build.
Current AI solutions are built by looking at patterns represented in well-annotated historical data and using feature engineering to build specific features for specific use cases.
The model that is produced is tailored to a specific use case – and can hardly be reused for another, even a similar use case.
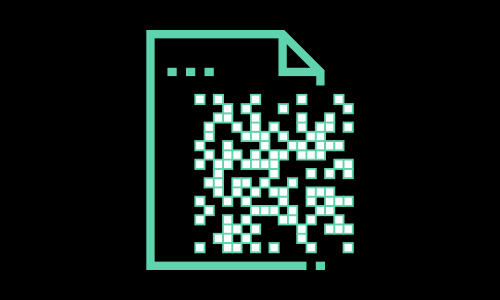
Lack of well-annotated data
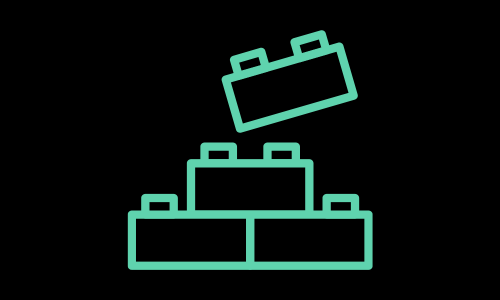
Long model build time
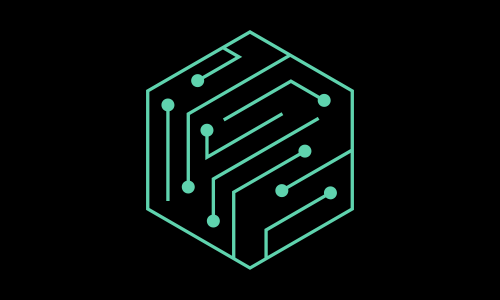
Blackbox AI that can't be explained
One model. Multiple use cases.
A shift towards flexible and reusable AI models. Amygda’s time series foundation models can be used for multiple use cases including smart maintenance, route optimisation, or even equipment life forecasting.
This means companies don’t have to wait for large amounts of data to be annotated in order for them to start seeing returns on their investment in AI.
By not relying on well-annotated historical information or labels, we remove the major blocker in scaling AI across your whole fleet of multiple use cases, enabling full fleet coverage for any equipment.
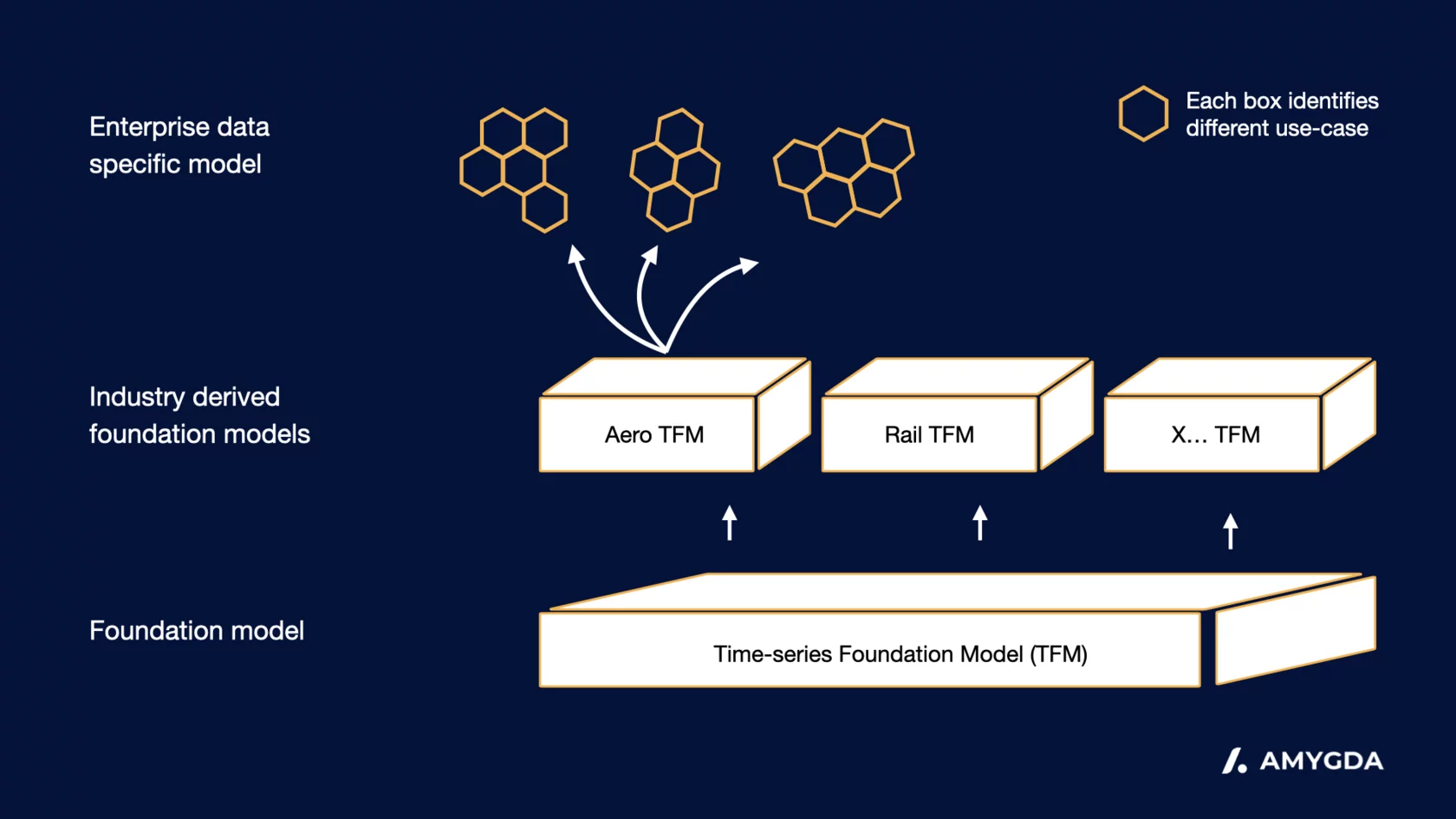
Benefits of this new approach
Amygda’s AI models overcome a major challenge in the lack of large sets of well-annotated data. This presents a significant advantage in real-world applications, as it eliminates the risk of equipment failure on critical assets.
Amygda’s models are built using unsupervised and semi-supervised techniques, with further development focusing on self-supervised learning. This approach has reduced reliance on well-annotated datasets, paving the way to addressing novel, diverse and less understood use cases.
AI projects consume a long time in data engineering and rebuilding each time from scratch. By removing this rebuild we can drastically speed up time to production.
Amygda’s models are built with a focus on transfer learning. This has enabled reusability as Amygda’s time series foundation models can be used for multiple use cases, including smart maintenance, route optimisation, and equipment life forecasting. This approach has sped up data centric aspects from months to days, with a vision to reduce the time further to minutes.
Just as we prefer working with honest and trustworthy colleagues, collaboration with AI requires a similar level of trust. Explainable and Trustworthy AI is a prerequisite for any successful embedding of AI in operational processes.
At Amygda, solutions are built to not only provide an interpretable output but also deliver insights into the factors and reasoning behind the decision making process. This is done by the use of explainability algorithms that sit on top of our underlying solutions.
Why now?
Why work with us?
Get ROI across your whole fleet
As a fleet operator, you own multiple assets and equipment from several different OEMs. Our AI platform works across any equipment in your fleet, irrespective of its type or age. So you can get whole fleet coverage for any use case.
Embed AI across the business
Scaling AI improves productivity. Multiple teams managing fleets of assets can now benefit from Amygda's solutions. Our AI platform is scalable and enables business leaders to embed AI in various use cases across the business.
Faster time to value
Equipped with billions of data points from 100s of different equipment types, Amygda is already demonstrating a reduction in time to value from months to weeks, and in some instances, days. So you can demonstrate business value faster.
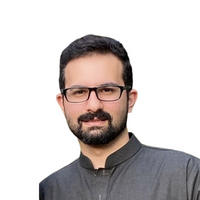
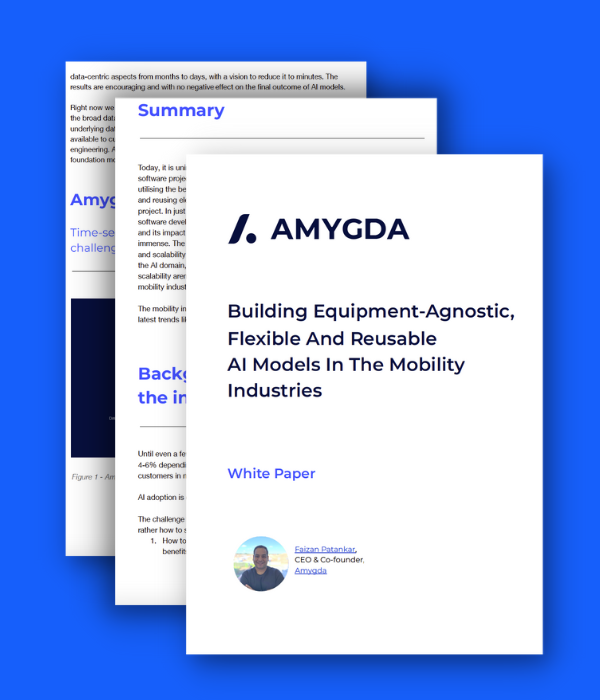