This blog is co-produced by Amygda and Rheinberry, a specialist Aviation Security and Airport IT consultancy.
A non-traditional approach to overcoming the challenges of equipment failure and the lack of sufficient data to train traditional AI models for monitoring airport baggage and security systems.
Introduction
Airports, as bustling transport and economic hubs, shoulder the responsibility of efficiently managing a constant influx of people and goods. It’s a complex ecosystem that demands seamless integration of new security equipment, technology, and people.
In this blog, we explore the pressing challenges in the airport baggage systems sector, shedding light on the transformative potential of Generative AI for airports, airlines, and passengers – with reliability as the cornerstone of the airport experience.
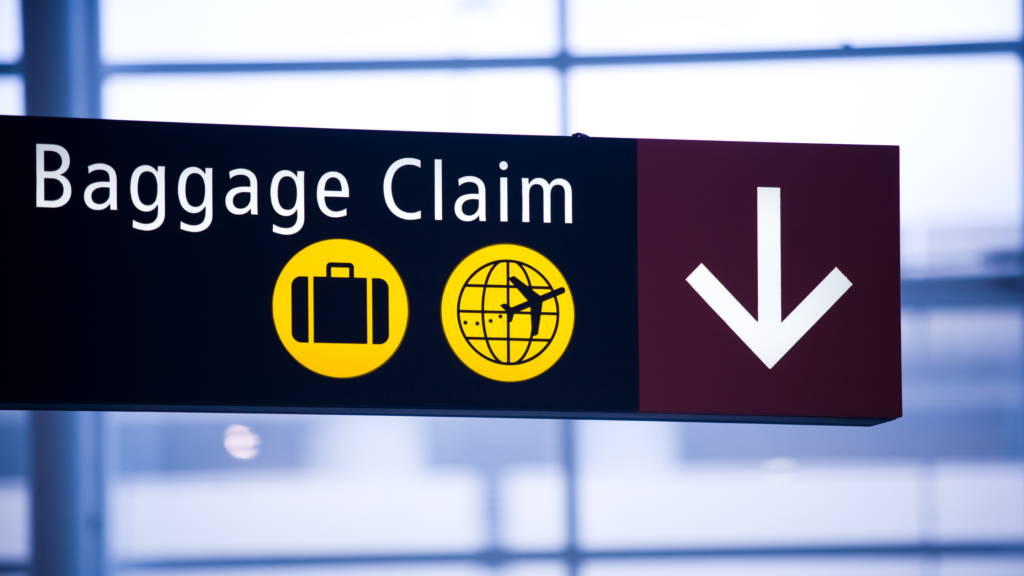
Challenges in Airport Baggage and Security Systems
From the intricacies of screening processes and baggage handling to the demand for real-time prohibited item detection, airports face a myriad of challenges that necessitate a paradigm shift in security methodologies.
Equipment Failure: A Bottleneck in Baggage and Security Systems Infrastructure
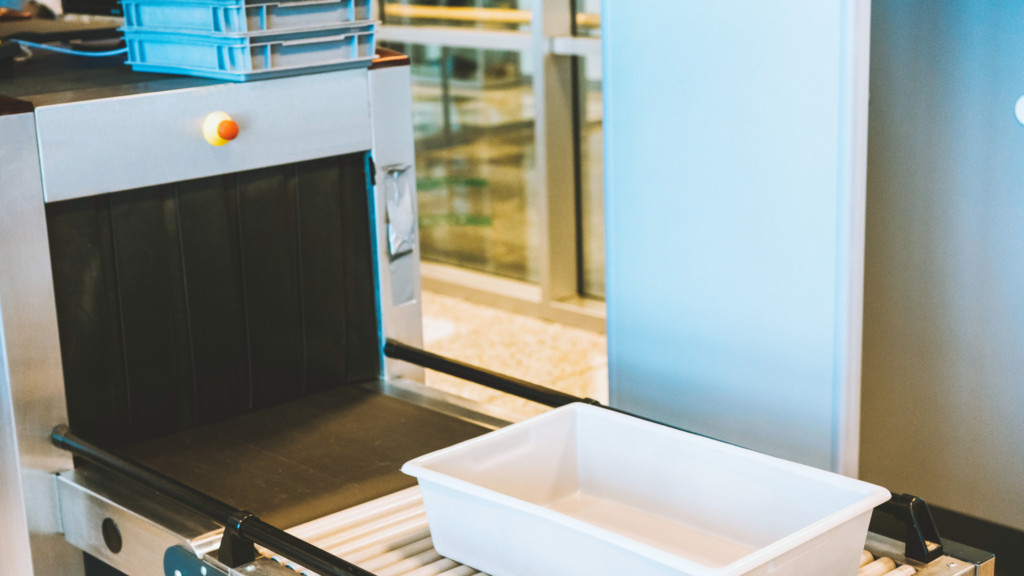
Airports rely on complex equipment to implement and manage security and provide airport baggage handling. Primarily using X-ray machines for baggage scanning and mm-wave or metal detectors for people screening, these essential devices face frequent failures, hampering overall security.
Some equipment is reaching its end of life and will soon be superseded by newer equipment. Furthermore, planned maintenance occurs on strict schedules. Where maintenance teams and engineers are located remotely, this can make unplanned maintenance problematic.
Airports are currently in the process of upgrading their security equipment, but therein lies one of the challenges – there is no historical data on those new machines, which makes the diagnosis of a failure harder. Alongside this are continuous updates to baggage handling systems often comprised of multiple conveyors, sorters and diverters each with motors, controllers (PLCs) and sensors.
Failure in airport baggage and security systems means delays for passengers and airlines, disruption to air traffic and high maintenance costs. This also translates to low satisfaction for the airport, reputational damage and loss of income.
Lack of Data: Amplifying the Cost of Unpredictability
The complexity of baggage and security system failures, often linked to unpredictable issues, results in a high cost of maintenance to airports, as maintenance technicians may be located remotely, there is no data on root cause analysis or an indication of how long the repair might take. The costs associated with unplanned maintenance can become a significant challenge, impacting the airport’s operations and finance planning.
Improving access to maintenance data and providing well-understood descriptions of the data for both OEMs and airport operators is fundamental to improving maintenance strategies. Addressing the root causes of equipment failure necessitates a collaborative approach that integrates Generative AI to unlock predictive insights and transform the current landscape of airport security.
Overcoming Airport Baggage and Security System Failures with Generative AI
Generative AI is emerging as a game-changer, providing innovative solutions for managing equipment and systems, troubleshooting in real-time, and improving the experience of passengers and airlines at airports. The real-time decision support offered by Generative AI solutions can assist airport security equipment maintainers in making swift and informed decisions. One such solution is Amygda’s AI Assist. It turns hidden and unstructured data into actionable steps at the fingertips of maintenance personnel.
Smart Maintenance for Baggage and Security Systems
Unlike traditional predictive maintenance, Generative AI doesn’t rely solely on predefined rules and historical occurrences. Instead, it leverages advanced models to generate synthetic data, offering a more nuanced understanding of potential issues. This innovative approach goes beyond minimising downtime and optimising maintenance schedules; it introduces a dynamic element, adapting to evolving scenarios and providing a more holistic and effective strategy for enhancing security operations while reducing costs.
Real-Time Anomaly Detection
Generative AI excels in real-time anomaly detection by continuously analysing live data from baggage and security systems. Whether it’s identifying equipment malfunctions before they cause failure, or helping technicians troubleshoot in real-time, Generative AI provides airport personnel with swift decision support. This capability enhances airport operations, reducing the risk of failures and ensuring a disruption-free environment for passengers and airlines.
Root Cause Analysis
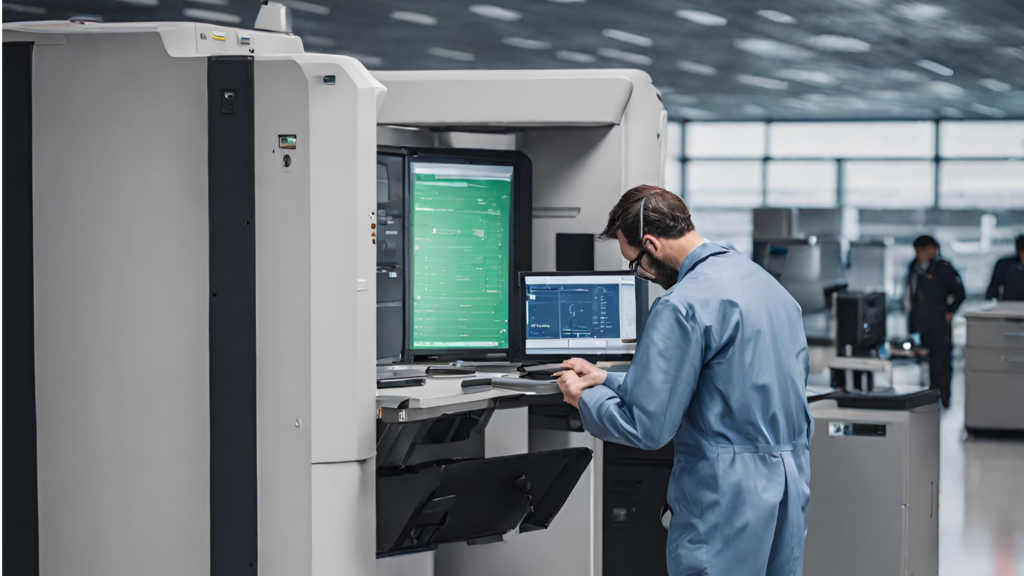
By meticulously analysing vast datasets encompassing historical maintenance records, real-time performance metrics, and patterns of equipment malfunctions, Generative AI brings a new level of sophistication to root cause analysis. Its ability to find intricate patterns and correlations enables the identification of potential triggers for equipment failures, empowering maintenance teams to address issues swiftly and effectively.
Overcoming the Lack of Data with Generative AI
Airports can overcome the challenge of the lack of data on new equipment when adopting Generative AI solutions for maintenance through several strategic approaches.
Reliable Synthetic Data Generation
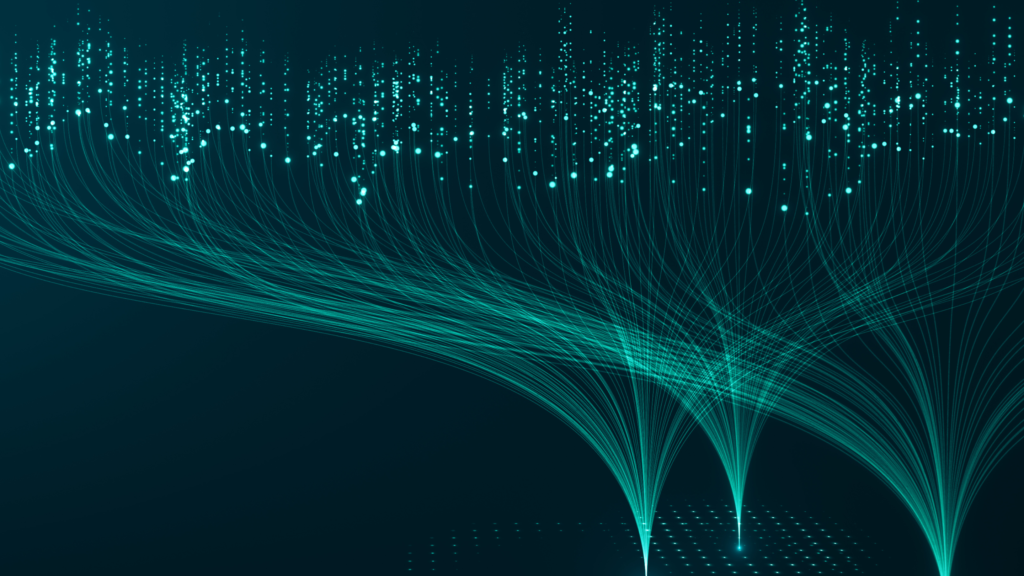
Generate synthetic data that mimics the expected behaviour of the new equipment and systems. This artificial dataset can be created based on the specifications and functionalities of the equipment and systems, providing AI models with a diverse range of scenarios for training.
Amygda uses advanced generative models to generate synthetic data in the aviation sector.
Parallel Data Collection
Conduct parallel data collection during the initial phases of deploying new X-ray machines. This involves collecting real-world data on equipment performance, failures, and maintenance needs. This data can be used to enhance Generative AI models and improve their accuracy over time.
Transfer Learning
Leverage transfer learning techniques, where pre-trained models on similar equipment or scenarios are fine-tuned for the specific characteristics of the new equipment. Amygda’s domain expertise, coupled with flexible and reusable AI models specifically built in the industry can be applied to airport baggage handling and security systems with minimal fine-tuning.
Continuous Monitoring and Feedback Loop
Implement a continuous monitoring system that tracks the performance of the new equipment. Establish a feedback loop where the AI model continually learns and adapts based on the incoming data, ensuring that it remains effective in the evolving operational environment.
By implementing a combination of these strategies, airports can mitigate the lack of data challenges and ensure that AI solutions for maintenance are well-equipped to handle the intricacies of new equipment. This proactive approach will lead to more accurate predictions, efficient maintenance practices, and overall improved operational resilience. Amygda has worked with airports on upgrading their baggage handling and security systems and is equipped to help them navigate the challenges of machine failures and lack of data through the application of explainable and trustworthy AI solutions.
Conclusion
Generative AI has the potential to be a transformative force in optimising baggage and security operations and maintenance at airports. From predictive maintenance to real-time anomaly detection and root cause analysis, its applications redefine how airports can optimise baggage and security systems, troubleshoot in real time, and enhance the overall experience for passengers and airlines.
To find out more about Amygda’s Generative AI for Airport Equipment and the use cases we
have worked on, contact us at [email protected]
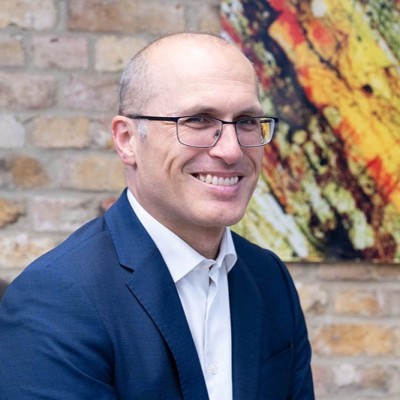
Richard Dempers – Founder and Director at Rheinberry, focusing on Aviation Security Open Architecture and integrating AI in airport operations.
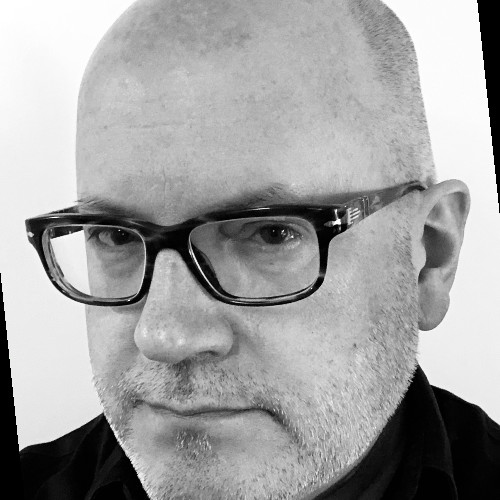
Joe Yaeger – Founding Partner at Rheinberry, focussing on IT and Security projects at airports.
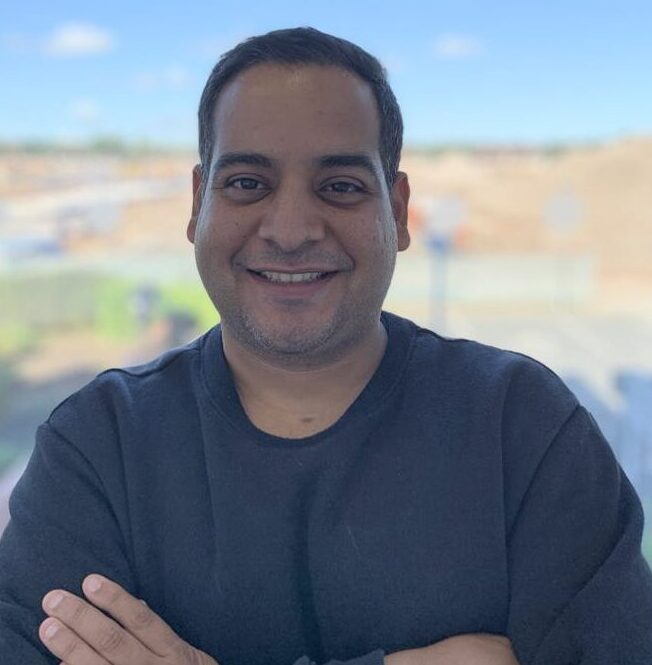
Faizan Patankar – CEO of Amygda, focussing on deploying AI in airport operations for smarter maintenance and operations of assets.