Introduction
Fleet management is a crucial aspect for any transport business, and monitoring the fleet’s performance is essential for improving efficiency, standardisation, and compliance. However, many businesses struggle with fleet-wide monitoring, particularly when working with multiple Original Equipment Manufacturers (OEMs) or when OEMs do not provide such services.
In this article, we’ll discuss the importance of fleet-wide monitoring for lower maintenance costs and higher return on investment (ROI) in the long term and how businesses can achieve these benefits even without OEM support. Because, as we have written in a previous post, OEM mandated maintenance is optimised for warranty claims, not uptime.
Fleet-wide monitoring for smart maintenance
Transport fleets generate a large amount and variety of data, and when looking at all this data without analysis and insight, it becomes unexplainable and meaningless. However, in order to make decisions and get useful insights, it is important to organise and visualise the respective data and trends within.
To illustrate this point, let us take the example of a large airline that operates a diverse fleet of aircraft. Even after collecting, analysing, and visualising all relevant data, it can still be a daunting task to manage such a large and complex fleet without a comprehensive fleet-wide visualisation platform that integrates all the necessary information.
Fleet-wide monitoring is crucial for optimising operations, enhancing safety, and increasing maintenance ROI, allowing businesses to monitor all their equipment, regardless of the OEM. By implementing a fleet-wide monitoring system, businesses can gain valuable insights into their fleet’s performance, improve decision making, and take proactive measures to improve efficiency and compliance, ultimately leading to better ROI in the long run.
Inefficient equipment usage can cripple a fleet’s productivity, leading to costly downtime and poor performance. By centralising all your data points, you’ll gain unparalleled insight into your fleet’s performance and be able to optimise it like never before. Fleet-wide monitoring offers a unified platform for data gathering and reporting.
One platform for your whole fleet
At Amygda, we ensure the coverage of multiple assets from any OEM, connecting all equipment in one platform, mapping assets and data in real time, allowing customers to make decisions faster.
From our conversations with customers, we understand that they find it difficult to utilise their own data from across the whole fleet to unlock value and reduce costs. With automated alerts that catch emerging issues before they become problems, you’ll keep your passengers moving and your engineers in the know.
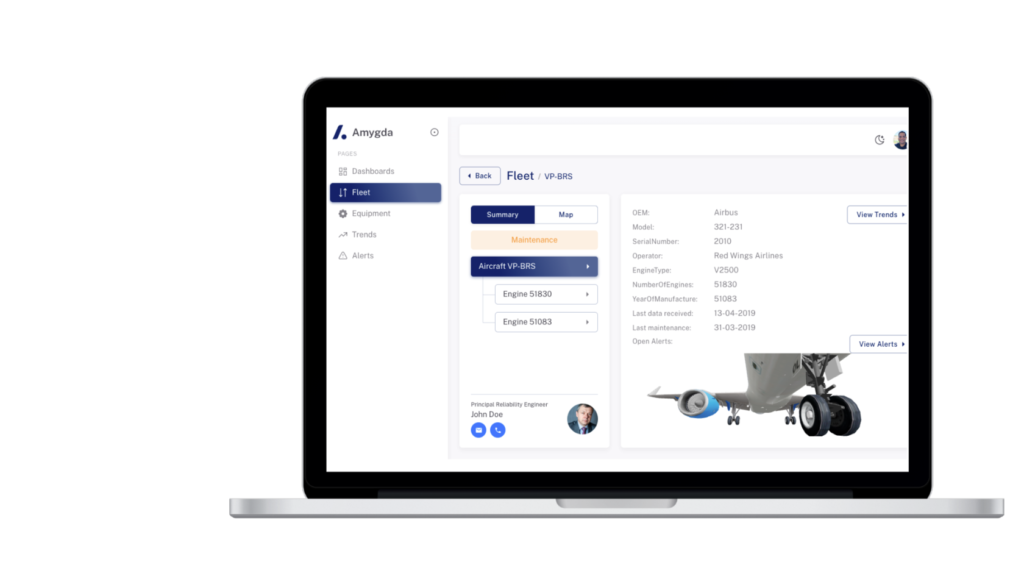
Amygda’s platform allows users to identify recurring issues and their root causes, predict issues based on historical data, such as previous maintenance events, and it offers a holistic view of the whole fleet in a single platform. Our customers can set rules, triage alerts, and get customisable workflows out of the box. As a result, fleet engineers can monitor all their equipment, implement changes quickly, get customisable workflows, and integrate with any ERP system.
There are various pitfalls for transport organisations who would like to utilise AI to optimise their operations and resources. Before embarking on a journey towards implementing AI for smart maintenance, organisations must answer a few key questions. First of all – what is the cost of the problem that smart maintenance will solve; how valuable is the solution to this problem to the business; what is the value difference between building a solution internally and buying one externally?
Assigning value/cost to a problem
At the heart of decision-making for businesses is determining the value versus the cost of solving a problem. To do this effectively, it’s crucial to assess the importance of the problem – this will have a direct impact on how much it’s worth spending to fix it. You also need to consider the potential benefits of solving the problem, as well as the potential risks or costs of not addressing it.
This requires taking into account issues such as the problem’s complexity, the availability of resources, and the potential trade-offs between different solutions. In order to quantify the problem and the benefits of solving it, we need to understand the nature of the problem and the root causes of it. Gathering data at this point and determining the impact of the problem is crucial to gain more insight and put a value on the problem.
A proactive approach to smart maintenance
We have spoken with multiple customers, and conducted thorough market research to find out how crucial it is for customers to solve problems when they arise. We have found that in asset heavy industries, such as aerospace and rail, the risk of neglecting a problem, such as in an aircraft hydraulic system, is much higher than taking care of it before failing, and paying a recurring fee for early detection.
In this particular case with a regional US airline, Amygda’s solution allowed the airline to confidently apply a proactive approach to maintaining their hydraulic system. Moreover, the airline could go back to their pump supplier with evidence of faulty pumps being fitted back on to aircraft after maintenance.
Finding how to assign value/cost to a problem in heavy asset transport industries is a challenging task, as it affects broader strategic and financial goals, but if we consider ROI as the main metric for a business, then the value of solving a maintenance problem early by far exceeds the cost.
Build vs buy solutions
Another question that companies might consider in these instances is whether to build a solution internally or buy one externally. Deciding whether to build or buy is a challenge with many potential pitfalls, as it depends on factors that are difficult to quantify and evaluate.
If we consider both situations in parallel – many companies choose to build internally if the cost of buying is higher, but determining these costs can be a risk in itself, as unexpected expenses, inefficiencies or lack of experience can often mean the actual budget is times higher than the planned budget.
When building internally, the company usually considers core competencies, intellectual property, how much customization this solution requires, and whether it can be applied to a general set of solutions. In the case of having internal core competencies, the obvious solution would be to build internally to leverage those strengths, but more resources might be required over time. Moreover, if the company has developed unique intellectual property that is critical to its operations, it may be more advantageous to build internally.
At the same time, buying externally comes with advantages, as more specialised knowledge would be available. For larger corporations, risk management is often a top concern, as a supply chain disruption can be costly. In such cases, having multiple suppliers can help mitigate this risk.
At Amygda, we have spent more than three years as a team perfecting our solution and tailoring it to the needs of our customers. We have deep knowledge and expertise, as both our co-founder are ex-Rolls-Royce innovation leads with more than 15 years experience in data platforms. We have built a time series data platform that can be applied to multiple use cases within a business, and can be deployed within days, not months. This way, the business can access insights from day one, and reap the benefits of their investment straight away.
Watch this video and hear what Derek Bleyle, Product Manager at ClearObject, has to say about working with Amygda and implementing our solutions for ClearObject’s customers:
Managing models and the need for iteration
All algorithms have common classifiers that are iteratively changed to achieve the desired output. The iteration process in algorithms leads to a higher rate of accuracy, and it is fundamental to the design of certain algorithms, which can be scaled according to data size. Because of their potential to perform faster and better, these algorithms are at the forefront of machine learning applications.
Managing models and the need for iteration is a critical component of effective model development and deployment. In order to manage models considering the need for iteration, it is important to establish clear goals, define metrics, test and validate, and finally monitor performance in order to iterate further as needed.
Defining clear goals helps guide the model development process and ensure that the model is aligned with business objectives. Specific and measurable metrics are helpful to define and measure the performance of the model in order to test and validate. After validating, monitoring performance and comparing it to the metrics aids in identifying areas where the model may need to be updated or improved, and iterates as needed.
Find out more about the first foundation model specifically built for the transport industry.
Metrics to focus on on the business side and product side
According to a paper from Ruskin et al. on “Alarms, alerts, and warnings in air traffic control: An analysis of reports from the Aviation Safety Reporting System”:
“The overarching goal of a signal philosophy is to improve the controllers’ trust in the system and increase performance and efficiency. Controllers need to know the nature of the problem, the locations and altitudes of the involved aircraft or vehicles and the urgency of the problem. Signals should also be transparent, providing an indication of why the signal is being presented, the likelihood of the condition, and the urgency of the condition. Alarm flood can be avoided by inhibiting multiple alarms arising from the same deviation. A summary window can provide a list of current situations requiring a controller’s attention.”
At Amygda, we are constantly improving the accuracy rate and removing false negatives by testing models with real-world use cases and learning from the broad data. At each iteration, the time it takes to create representations in the underlying data keeps reducing, thus making it faster for users and customers to act on the signal/alert in a timely manner, which builds confidence in the accuracy of the performance and functionality of our models.