Aircraft health monitoring for systems embedded in the airframe and various LRUs like engines, avionics, and hydraulic systems have become increasingly crucial for safety and efficiency. Traditional rule-based systems have long been the backbone of predictive maintenance in aerospace.
However, the recent advancements of AI in aircraft maintenance are changing how we approach maintenance forecasting and overall fleet management. This article explores the limitations of rule-based systems and the step-change in the benefits of aerospace AI in predictive maintenance.
Rule-based predictive maintenance systems rely on human knowledge
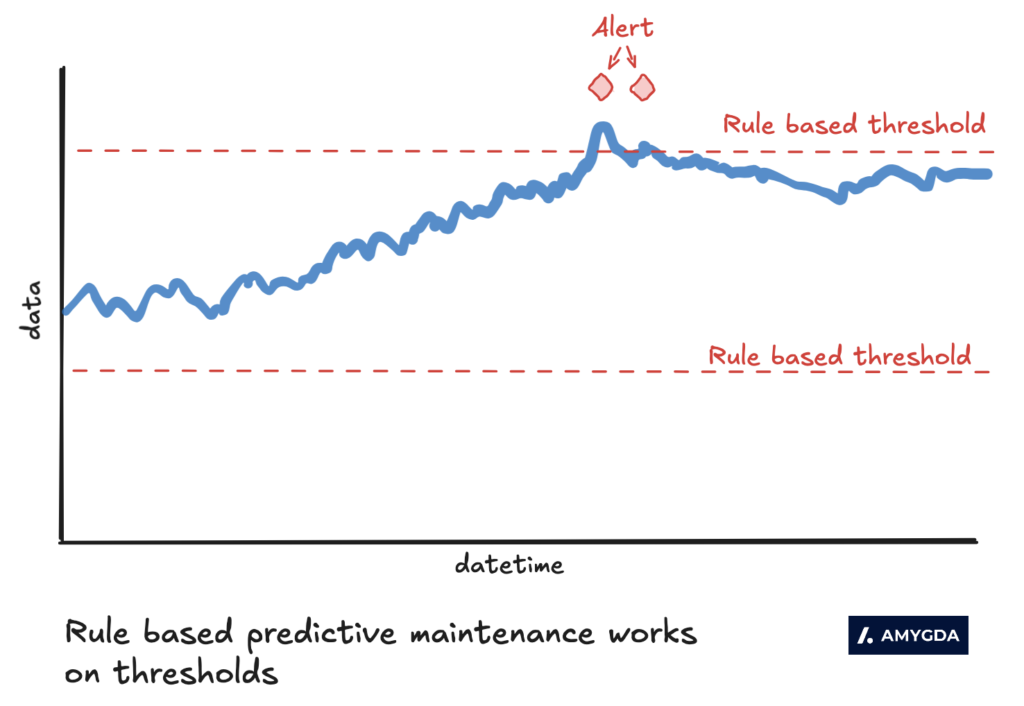
Rule-based systems are commonplace in predictive maintenance understanding. These systems are simple to understand and perform well in well-understood situations.
Rule-based systems involve setting up thresholds and monitoring each parameter for a breach of these thresholds.
For example, exhaust gas temperature (EGT) in degC should be between 600 and 700 degC. If the EGT goes above 700 degC, send an alert.
The same is applied to vibration, oil quantity, pressures, temperatures, etc.
These are theoretical and ‘design’ limits. They are created when the equipment is designed and manufactured.
But what happens when an aircraft is now flying in hot countries or through generally warmer temperatures? Is it really ‘not an issue’ when the temperature is 699 degC, and it is an issue if the EGT is 701 degC flying out of Saudi Arabia (a country with a hot climate)?
In rule-based systems for predictive maintenance, humans program the rule. A sort of IF….THEN….ELSE statement.
Introducing AI-based predictive maintenance systems
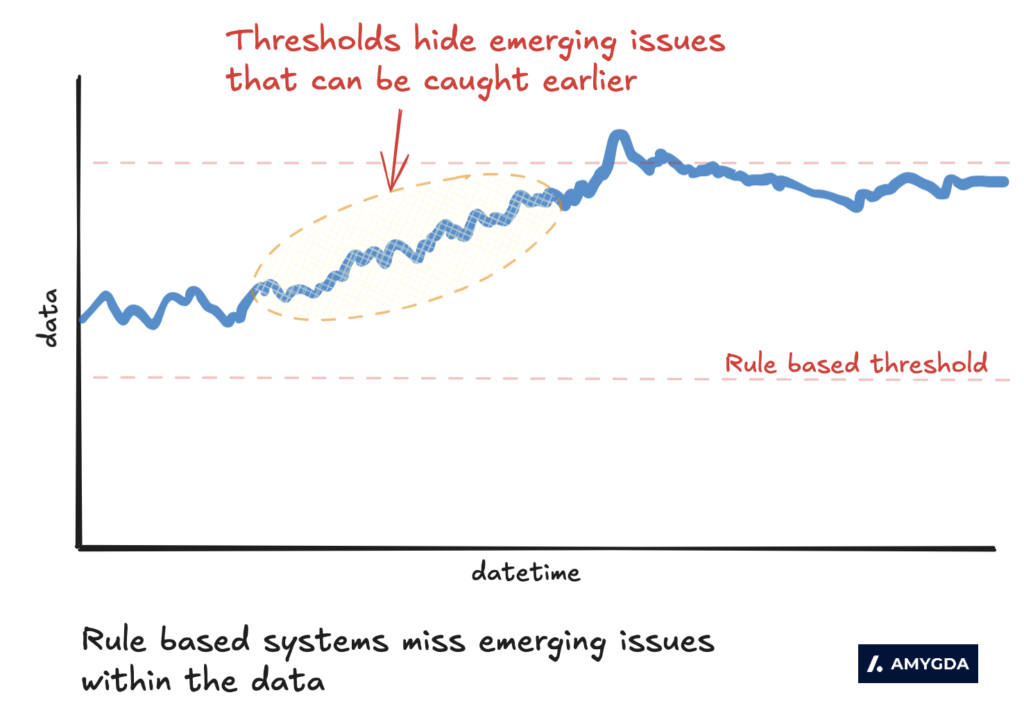
Conversely, AI-based predictive maintenance systems are flexible and adaptable to your operation. Constantly learning and adapting to ‘new normal’ conditions.
The AI learns from the data as it arrives. Learning and embedding knowledge with each new data point and with feedback from a human in the loop.
For example, using EGT again, an AI-based predictive maintenance system monitors multiple parameters and learns that it is the ‘rate of change’ in the EGT trend that matters more. And if the EGT trend, along with several other parameters moving in specific directions, are a cause for concern. Not just the individual parameter.
In this case, if the EGT has increased from 650 to 680 in the past 3 days, that’s a cause for concern even though the EGT is within the limits.
In an increasingly complex operation, having AI on all this data is like having 1000s of people watching the data and making decisions.
Unless you can hire 1000s of people, your choices are simple rule-based systems or AI-based predictive maintenance systems.
This also helps with monitoring your whole fleet, irrespective of the OEM, in one single platform. We call it a platform-agnostic MRO platform.
So is a rule-based predictive maintenance system bad?
No, those systems aren’t bad. They are after all built on human knowledge. We call this ‘known-known’ issues. i.e. We know how these equipment fail and we can write a code for it.
This covers 30-40% of the issues with equipment. The remaining 60% of the maintenance (for example in aviation) which is unscheduled is a result of issues that humans did not know could happen at the time that they have happened.
If you are creating a new service which provides availability or guarantees a high service agreement of equipment uptime (for example Rolls-Royce’s availability business model), then having as much coverage of the issues, both known and unknown is desirable.
After all, you do not want to run a service where you don’t know 50% of the time what is likely to happen with the aircraft.
P.S. We care a lot about known-known and known-unknown scenarios; we published a paper on using deep generative models for aircraft data for our research work with Loughborough University, UK.
AI in aircraft maintenance is important to cover the knowledge gaps
Engineering resources – technicians, mechanics, pilots, and more are all in shortage at airlines and the MRO sector. Whether you listen to Lufthansa, Boeing, or even McKinsey – the data points in one direction – a shortage of knowledge workers.
This is a result of ageing and retiring workforce, combined with those who left the industry (because of unchecked job losses) who are now in other jobs and don’t want to return to aviation. The shortage of workers in aviation and MRO is set to last until 2036-2040.
So this makes rule-based maintenance systems more challenging to deliver for a reliable service. Because you have to set the rules. And how many rules do you need to set?
It’s impossible to even know everything that can go wrong.
This is why AI-based predictive maintenance systems in airlines can complement the OEM solutions initially and cover the known-unknowns.
Whatever system you use, the result you are looking for is to provide a reliable journey for your customer.
What are the benefits of AI-based predictive maintenance systems?
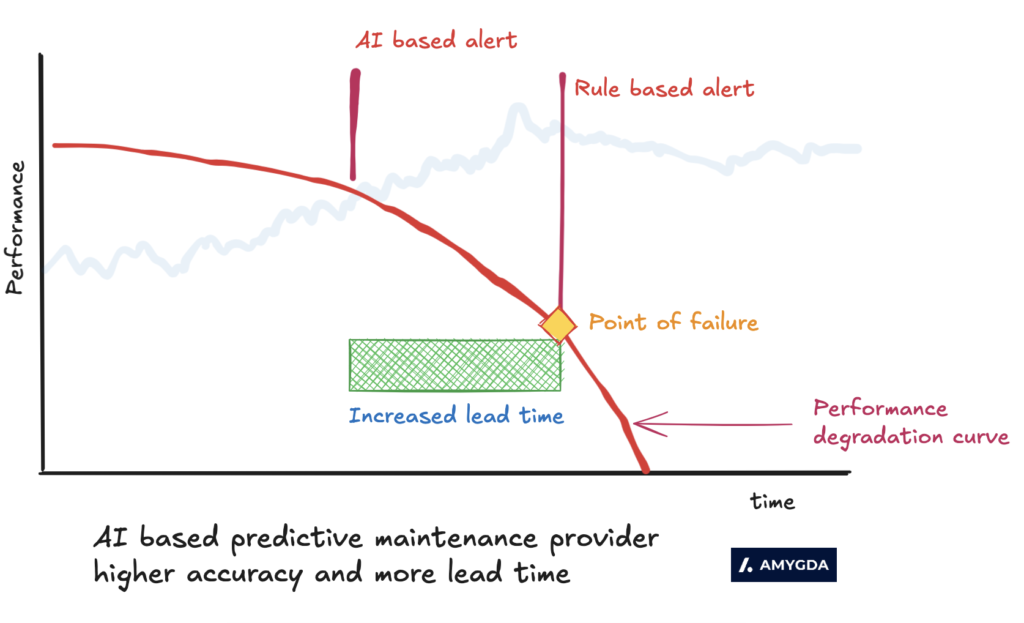
AI-based predictive maintenance is flexible; it learns from your operation which leads to better forecasts. Giving your engineers more confidence in the system.
Alerts generated by the AI system are from the source truth of data generated by the aircraft. It means that when an alert is generated it’s because there is a shift in data that either aligns with previous known issues or a new emerging issue. Both of these are important for maintenance and technical ops at airlines to be aware of.
More accurate forecasts and increased coverage mean the overall maintenance burden on engineers reduces as they focus on aircraft systems that need maintenance, rather than manually reviewing it all.
Having an AI-based aircraft predictive maintenance system is like having 1000s of engineers looking at data.
The shift from rule-based systems to AI in aircraft maintenance is not just beneficial – it’s essential.
How do we test AI-based predictive maintenance systems?
At Amygda, we focus on AI-based systems using an AI engine that has now seen over 3TB of proprietary equipment data.
Amygda’s AI platform automates data analysis, generating real-time fleet health forecasts and providing early, accurate warnings of potential problems. Making aircraft maintenance operations smarter and keeping your operations reliable, boosting dispatch reliability across your entire fleet.
Here’s how it works:
- Quick setup: The AI learns from your data in days, not months. It consistently achieves accuracy rates of 90%+ across various aircraft types and operational conditions, as validated through extensive testing and real-world deployments.
- Works with any equipment: No matter what planes or parts you use, our system can handle it.
- Easy to understand: The AI explains its findings clearly, so your team can make good decisions.
- Automatic problem-spotting: The AI watches your aircraft systems 24/7. It finds issues early by looking at single factors and how different parts work together.
- Focused maintenance: When the AI spots a potential problem, it alerts your team and shows them exactly where to look. This saves time and helps fix issues before they become serious.
- User-friendly: Your engineers can easily use the system to find the root cause of problems.
Whether you’re looking to optimise your current maintenance processes or increase the coverage of your approach to aircraft health monitoring, explore AI-based solutions as a crucial step towards future-proofing your maintenance operations.