What are foundation models and what is their application so far?
The amount of data produced today, by both humans and machines, considerably exceeds the capacity of humans to understand and make complex decisions on that data. This is where Artificial Intelligence (AI) makes it possible to comprehend and make sense of this data.
In 2021 a new concept emerged in AI when a group of researchers from Stanford University published a paper in which they introduced the concept of Foundation Models. According to the Stanford Center for Foundation Models (CRFM), foundation models are a new successful paradigm for building AI in which one model is trained on a huge amount of data and adapted to many applications.
Essentially, they are like large AI models trained on enormous quantities of unlabeled data, usually through self-supervised learning.
Foundation models lead to improved performance, as they have shown a significant improvement in the performance of various NLP tasks like machine translation, sentiment analysis, and language modelling.
Moreover, they can learn from large amounts of data and generalise well to new tasks and domains without requiring extensive additional training.
For example, the model behind ChatGPT is a foundation model. Some other models include BERT, MAE, and DALLE-E.
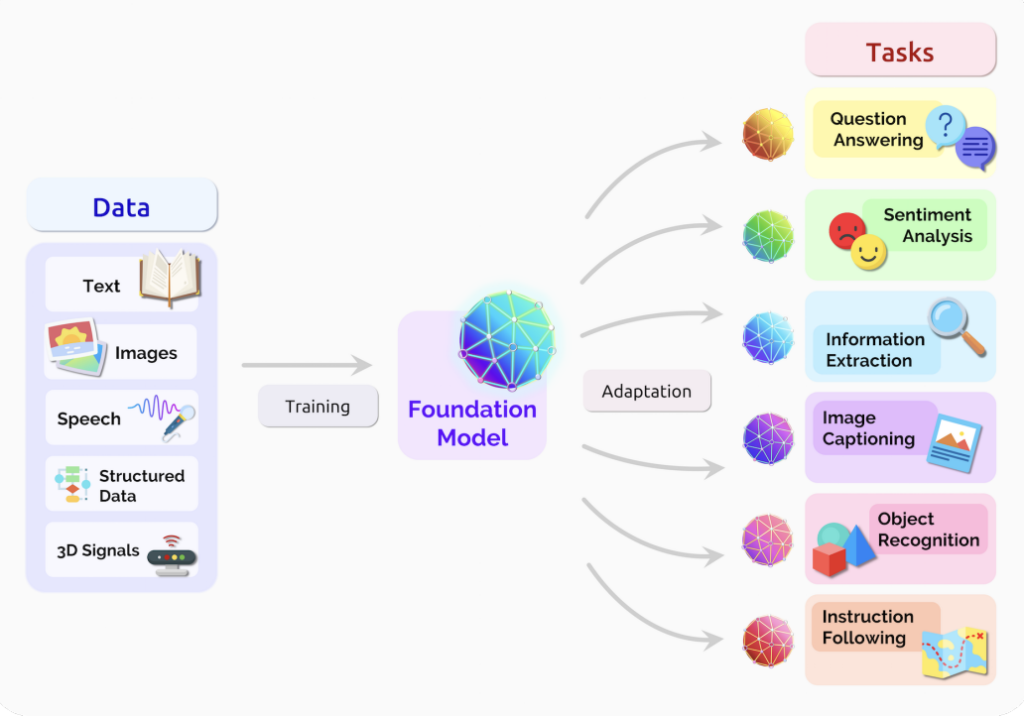
Figure 1. Source: “On the Opportunities and Risks of Foundation Models”
Foundation models in transport industries are yet to be established
There are several opportunities for foundation models to be used in the transportation industry.
Foundation models on maintenance data
This is going to be an exciting area of development for AI in the transport industry. For example, aircraft maintenance logs contain lots of information that is unstructured and proprietary. We can imagine this data being parsed through a large language model (LLM) like the one used by ChatGPT. Maintenance information can be structured and queried to find instances where an event has happened before, or even build a recommendation engine based on what was previously identified as the root cause.
Time series foundation models on sensor data
Another application of foundation models is on sensor data. Trains and planes are equipped with hundreds of sensors that are acquiring data and storing it in central databases. Time series foundation models could be really beneficial for customers in transport industries where well annotated or labelled data is not widely available.
Amygda is taking an approach to building the time series foundation model for transport industries.
Challenges of adopting foundation models in transport industries
Even though the opportunities and advantages of implementing foundation models are wide, there are many challenges that need to be addressed. The application of foundation models remains limited to a couple of fields, and there aren’t new ventures or projects that are trialling the use of foundation models in decision support systems in the transport sector.
There are several reasons why they may not gain wide and quick acceptance in the transport industry:
The biasness issue
Foundation models are trained on large datasets, which may contain biases. These biases can be reflected in the model’s output, leading to inaccurate or unfair results.
Lack of interpretability
Foundation models are complex, and it can be difficult to understand how they make predictions. This lack of interpretability can make it hard to diagnose errors or improve the model.
Dealing with drifts
Foundation models are typically pre-trained on large datasets and then fine-tuned on specific tasks. However, as new data becomes available, it may be necessary to continue training the model to maintain its performance. Continual learning is a challenging problem, and current methods require careful design and optimization.
Overall, while foundational models offer many benefits in natural language processing, they may not yet be widely adopted in the transport sector due to various challenges related to computational resources, training data, model interpretability, deployment complexity, and fine-tuning.
Amygda’s innovative vision of bringing foundation models to aerospace and rail
At Amygda, we began with the vision of building flexible, reusable AI models that could be applied to different use cases on sensor data in the transport industry.
AI models that could self-understand the underlying representations in data. The underlying representations could be various patterns, identifying emergence or creating homogeneous cohorts from the data. It could be used for smart maintenance, finding optimised routes, or even forecasting the next steps in the life of the equipment.
These AI models will replace the use-case-specific models that have dominated the industrial analytics landscape.
Amygda has been training models on a broad set of unlabeled raw data from various types of equipment. These models are built using unsupervised and semi-supervised techniques and further development is focussed on transfer learning. This has sped up data-centric aspects from months to days, with a vision to reduce it to minutes. The results are encouraging and with no negative effect on the final outcome of AI models.
What is the future looking like for the intersection of foundation models with the transport industry?
Foundation models have thawed the AI winter and the speed of change is incredibly exciting. It’s a small sample size, but speaking to our clients and folks we engage with – there’s a real need to speed up projects and think about scaling AI beyond narrow, specific use-cases.
Faizan Patankar, CEO of Amygda
Unlike large text and image data sets which are publicly available, working with equipment sensor data has the added challenge of getting access to the data in the first place.
Amygda is already showing the value of training on large data and the benefits of self-supervised and unsupervised learning.
This does require a change in how transport industries think about AI adoption. Mainly the shift from supervised learning techniques to semi-supervised and self-supervised learning; and becoming comfortable with the speed at which AI is going to change the efficiencies in the transport industry.
Summary
By 2025, enterprise AI will be entirely run on AI models that understand the underlying data representation and can solve multiple-use cases. Like a general-purpose sensor data model that is pre-trained to find data representations and structure data by merely fine-tuning. This is already happening with the language models where LLM foundation models have replaced use-case-specific NLP models. Obviously, these models can’t deal with sensor data and tabular formats, etc.
This requires AI models that are reusable and flexible towards different use cases and data formats in the engineering domain with sensor data, and an infrastructure that can operationalise these models for several different data volumes, velocity, and veracity.
Contact us
Drop us a line on [email protected] to chat to us about how we can leverage our existing solutions for your fleet of assets.